
Are advisory apps a solution for collecting Big Data?
By Jens Andersson, Jordan Chamberlin and Peter Craufurd
NAIROBI (Kenya) — Agronomic researchers face several challenges and limitations related to data. To provide accurate predictions and useful advice to smallholder farmers, scientists need to collect many types of on-farm data; for example, field size, area devoted to each crop, inputs used, agronomic practices followed, incidence of pests and diseases, and yield.
These pieces of data are expensive to obtain by traditional survey methods, such as sending out enumerators to ask farmers a long list of questions. Thus collected data is often restricted to a particular geographical area and may not capture key factors of production variability, like local soil characteristics, fertilizer timing or crop rotations.
As a result, such datasets cannot deliver yield predictions at scale, one of the main expectations of Big Data. Digital advisory apps may be part of the solution, as they use crowdsourcing to routinize data collection on key agronomic variables.
The Taking Maize Agronomy to Scale in Africa (TAMASA) project has been has been using mobile apps in research on how to provide more site and farmer specific agronomic advice to farmers through agro-dealers, extension workers and other service providers.
At CIMMYT, one of the research questions we were interested in was “Why are plant population densities in farmers fields usually well below recommended rates?” From surveys and yield estimates based on crop-cut samples at harvest in Ethiopia, Nigeria and Tanzania, we observed that yields were correlated with plant density.
We thought that farmers may buy too little seeds for their maize fields. One possible reason for this could be that farmers do not know the size of their maize field. Another reason could be that farmers and agro-dealers may not know how many seeds are in a packet, as companies rarely indicate it and the weight of each seed variety is different. Or perhaps farmers do not know what plant population density is best to use. Seed packets sometimes suggest a sowing rate but this advice is rather generic and assumes that farmers apply recommended fertilizer rates. However, farmers’ field conditions differ, as does their capacity to invest in expensive fertilizers.
We therefore developed a simple app, Maize-Seed-Area. It enables farmers, agro-dealers and extension workers to measure the size of a maize field, to generate advice on plant spacing and density, or to calculate how much seed to buy. It can also provide information on seed varieties available at markets nearby.
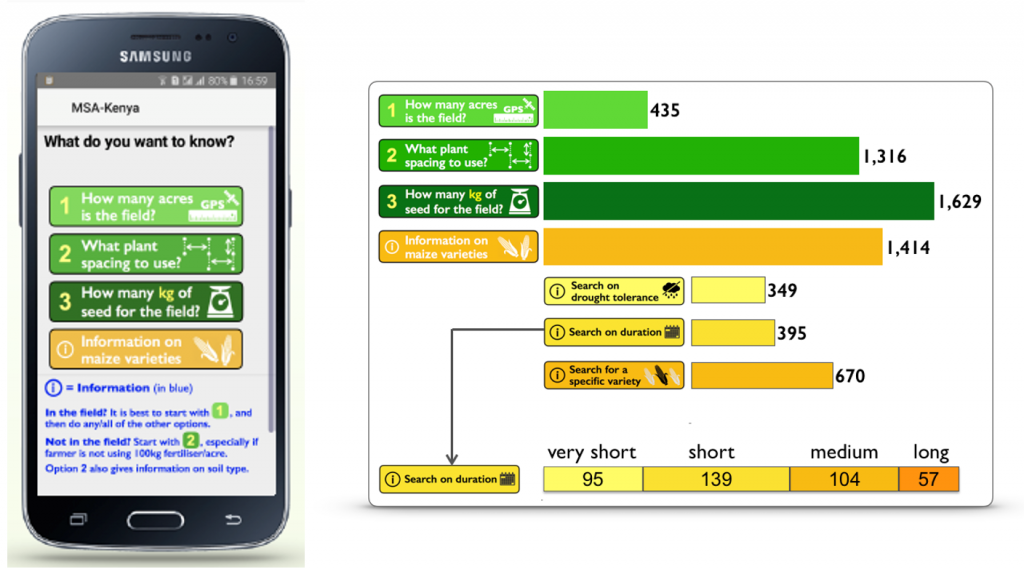
View of the interface of the Maize-Seed-Area app on mobile phones and tablets.
Maize-Seed-Area was developed using the Open Data Kit (ODK) format, which allows offline data collection and to submit it when internet connection becomes available. In this case, the app was also used to deliver information to the end users.
Advisory apps usually require some input data from farmers, so advice can be tailored to their particular circumstances. For example, Farmers might need to provide data on the slope of their field, previous crops or fertilizer use in order to generate a plant density advice. In addition to such input data, some other information may be collected through the app, such as previous seed variety use. All this data entered by the user, which should be kept to a minimum, is routinely captured by the app and retrieved later.
Hello, Big Data!
As the app user community grows, datasets on farmer practices and outcomes grow as well. In this case, we can observe trends in real time, for instance on the popularity of different maize varieties.
In a pilot in western Kenya, in collaboration with Precision Agriculture for Development (PAD), some 100 agro-dealers and extension workers used the app to give advice to about 2,900 farmers. Most of the advice was on the amount of seed to buy for a given area and on the characteristics of different varieties.
Data showed that the previous year farmers grew a wide range of varieties, but that three of them were dominant: DK8031, Duma43 and WH505. Collected within just 2 months prior to planting, this data became available as soon as the users connected to the internet. Most of the them have kept the app for future use.
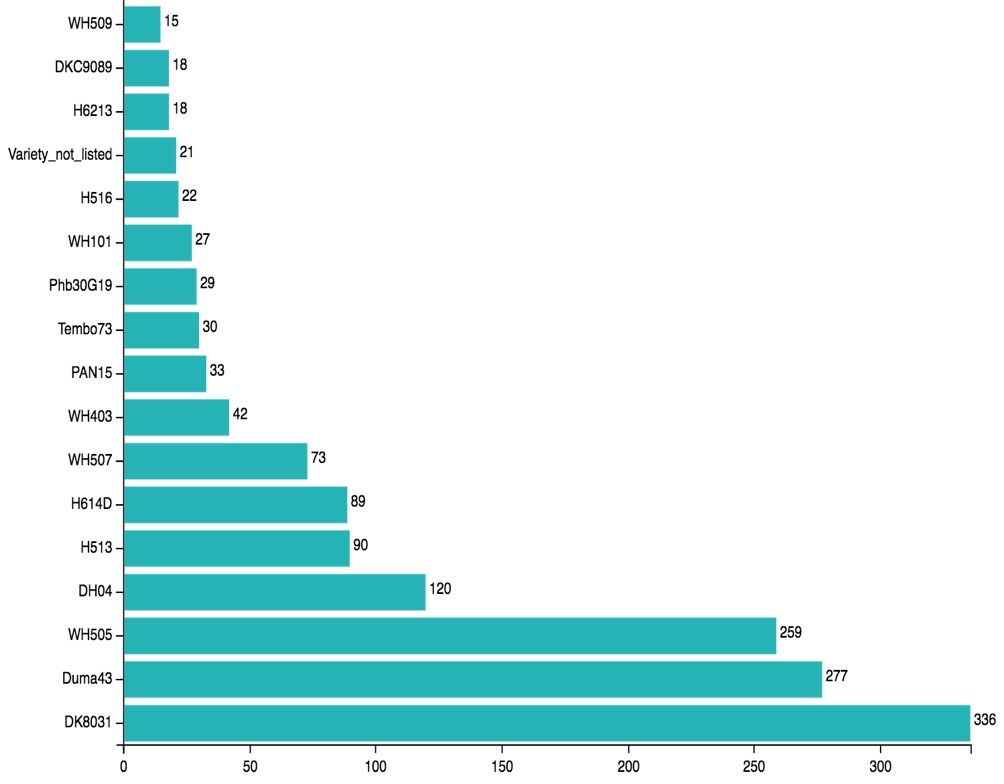
Preferred variety of maize of farmers advised with Maize-Seed-Area app. in western Kenya (Bungoma, Busia, Kakamega and Siaya counties), February-March 2018.
A phone survey among some 300 of the farmers who received advice found that most of them anticipated to do things differently in the future, ranging from asking for advice again (37 percent), growing a different maize variety (31 percent), buying a different quantity of seed (19 percent), using different plant spacing (18 percent) or using more fertilizer (16 percent).
The Maize-Seed-Area pilot shows that advisory apps, when used widely, are a major source of new Big Data on agronomic practices and farmer preferences. They also help to make data collection easier and cheaper.
Jordan Chamberlin, Spatial Economist at CIMMYT, will give his insights on best practices on electronic data capture on October 4, 2018.